Pandas and NumPy for Beginners
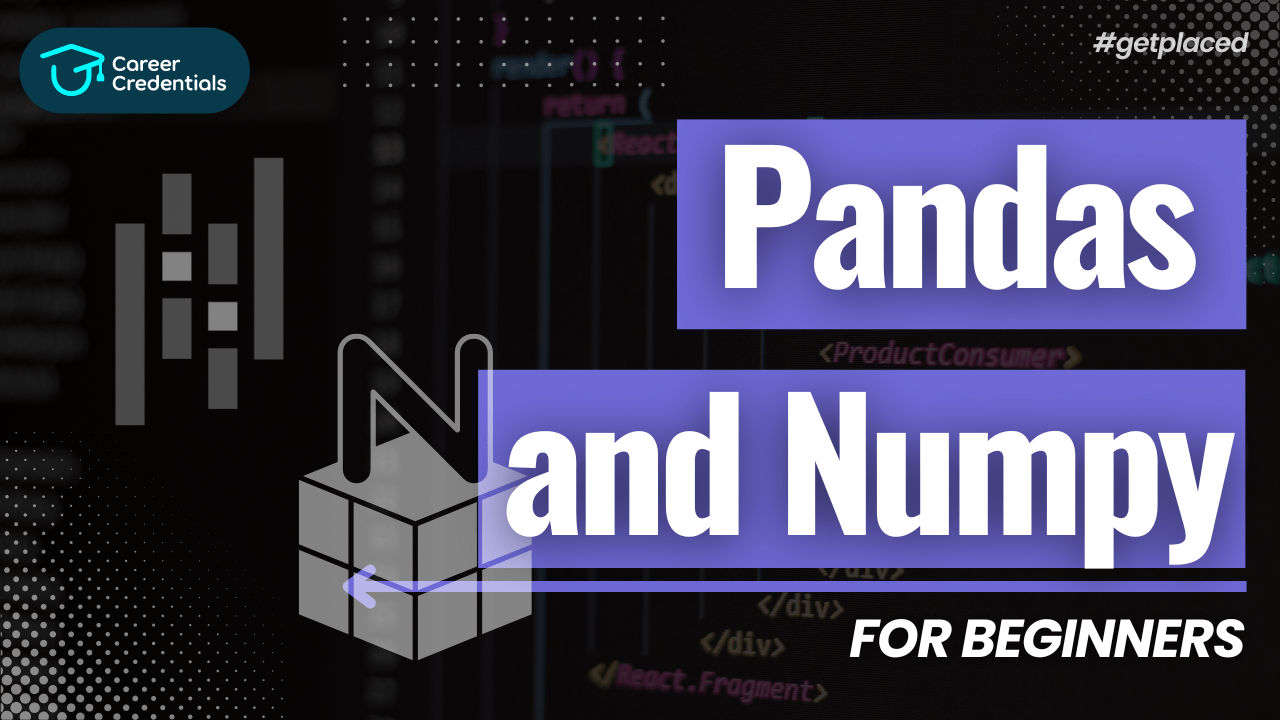
When diving into the world of data science and Python, two libraries you will undoubtedly encounter are Pandas and NumPy. These libraries are essential tools for data manipulation and analysis, and mastering them will greatly enhance your ability to work with data. This blog aims to introduce beginners to these powerful libraries, showcasing their functionalities, similarities, and differences, while providing practical examples to get you started.
Introduction to Pandas
Pandas is a widely-used open-source library designed for data manipulation and analysis. Its goal is to be the most powerful and flexible open-source tool for data analysis, and it has certainly achieved that goal. At the heart of Pandas is the DataFrame, a two-dimensional, size-mutable, and potentially heterogeneous tabular data structure with labeled axes (rows and columns). Think of it as a highly sophisticated spreadsheet in Python.
Key Features of Pandas
- DataFrames: Central to Pandas, DataFrames are structured like tables or spreadsheets with rows and columns, both having indexes. This structure allows for easy data manipulation and analysis.
- Handling Missing Data: Pandas has built-in functionalities to handle missing data efficiently.
- SQL-like Operations: Many SQL functions have counterparts in Pandas, such as join, merge, filter, and group by.
- Data Transformation: You can easily transform and reshape your data with various built-in functions.
Installing Pandas
If you have Anaconda installed, Pandas may already be included. If not, you can install it using the following commands:
conda install pandas
Alternatively, if you're using pip, you can install it with:
pip install pandas
Getting Started with Pandas
Before using Pandas, you need to import it into your Python environment. Typically, it is imported with the abbreviation pd
:
import pandas as pd
Introduction to NumPy
NumPy, short for Numerical Python, is a fundamental package for numerical computation in Python. It provides support for large multi-dimensional arrays and matrices, along with a collection of mathematical functions to operate on these arrays.
Key Features of NumPy
- ndarrays: NumPy arrays, or ndarrays, are more flexible and efficient than Python lists. They can have any number of dimensions and hold a collection of items of the same data type.
- Fast Element Access: Accessing and manipulating elements in a NumPy array is faster compared to Python lists.
- Vectorized Operations: NumPy allows for vectorized operations, enabling mathematical operations to be performed on entire arrays without the need for explicit loops.
Installing NumPy
Similar to Pandas, you can install NumPy using either conda or pip:
conda install numpy
Or with pip:
pip install numpy
Getting Started with NumPy
Before using NumPy, import it into your Python environment. It is usually imported with the abbreviation np
:
import numpy as np
Working with NumPy Arrays
NumPy arrays (ndarrays) are the foundation of the NumPy library. They can be one-dimensional (vectors) or multi-dimensional (matrices). Here are some examples to illustrate their usage.
Creating NumPy Arrays
To create a one-dimensional ndarray from a Python list, use the np.array()
function:
list1 = [1, 2, 3, 4]
array1 = np.array(list1)
print(array1)
Output:
[1 2 3 4]
For a two-dimensional ndarray, start with a list of lists:
list2 = [[1, 2, 3], [4, 5, 6]]
array2 = np.array(list2)
print(array2)
Output:
[[1 2 3]
[4 5 6]]
Operations on NumPy Arrays
NumPy arrays allow for various operations such as selecting elements, slicing, reshaping, splitting, combining, and performing numerical operations like min, max, mean, etc. For example, to reduce the prices of toys by €2:
toyPrices = np.array([5, 8, 3, 6])
print(toyPrices - 2)
Output:
[3 6 1 4]
Pandas Series and DataFrames
Pandas Series
A Series is similar to a one-dimensional ndarray but with additional functionalities. For instance, you can label the indices, which is not possible with ndarrays. Here’s an example of creating a Series with default numerical indices:
ages = np.array([13, 25, 19])
series1 = pd.Series(ages)
print(series1)
Output:
0 13
1 25
2 19
dtype: int64
You can customize the indices using the index
argument:
series1 = pd.Series(ages, index=['Emma', 'Swetha', 'Serajh'])
print(series1)
Output:
Emma 13
Swetha 25
Serajh 19
dtype: int64
Pandas DataFrame
A DataFrame is a two-dimensional labeled data structure with columns of potentially different types. Here’s how to create a DataFrame using a list of lists:
dataf = pd.DataFrame([
['John Smith', '123 Main St', 34],
['Jane Doe', '456 Maple Ave', 28],
['Joe Schmo', '789 Broadway', 51]
], columns=['name', 'address', 'age'])
print(dataf)
Output:
name address age
0 John Smith 123 Main St 34
1 Jane Doe 456 Maple Ave 28
2 Joe Schmo 789 Broadway 51
You can change the row indices to be one of the columns:
dataf.set_index('name', inplace=True)
print(dataf)
Output:
address age
name
John Smith 123 Main St 34
Jane Doe 456 Maple Ave 28
Joe Schmo 789 Broadway 51
Conclusion
Understanding Pandas and NumPy is crucial for any aspiring data scientist. NumPy provides the fundamental building blocks for numerical computations, while Pandas builds on top of these blocks to offer more sophisticated data manipulation tools. Mastering these libraries will empower you to handle, analyze, and visualize data effectively.
Whether you're a beginner or looking to deepen your knowledge, practicing with real-world data sets and exploring the extensive documentation for these libraries will further enhance your skills. Happy coding!